A Guide to Deep Learning in Quantitative Finance
Artificial intelligence, and in particular machine learning, has received a tremendous amount of attention in recent years. It has shown outstanding performance to solving a wide variety of tasks from almost all fields of science. The media attention has mainly focused on computer vision and language processing, but machine learning also shows great potential in the field of quantitative finance. Application areas include algorithmic trading, risk management, economic impact studies, asset allocation, and more.
In this paper, we explore the application of machine learning to quantitative finance. Typical quant finance applications depend on vast amounts of economic data with complex relationships which are hard to grasp by humans or traditional quantitative finance approaches. Machine learning has tremendous potential here, producing results far superiour to traditional methods. In particular, machine learning can detect and exploit complex relationships in the data which are neglected by - or are even unknown to - standard quantitative finance methods today. Candlestick chart with two moving average indicators, which can be used for deep-learning based algorithmic trading strategies.
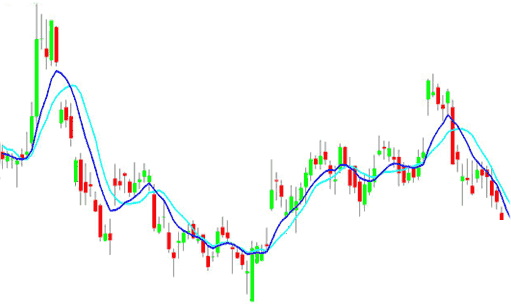
- Introduction to machine learning
- Application areas in quant finance
- Overview of machine learning architectures
- Superiour performance compared to traditional methods
- Insights into learner design
- Regressions, random forests, deep neural nets
- Recurrent neural networks (RNNs), long short term memory models (LSTMs), deep believe networks (DBNs)